Data-driven strategies’ true impact in strengthening business growth cannot be underestimated, more so during these very uncertain times. For almost a decade data has been a buzzword most were familiar with, but few had constructively leveraged it.
Data-Driven Strategies can provide you with a competitive edge in uncertain market:
To say these are uncertain times would be an understatement. Accepted conventions are being challenged and the way ahead cannot be summarized with annual projections. Around the world, the level of uncertainty related to the pandemic is high and unprecedented. According to an IMF report published in 2020, the level of uncertainty experienced by the markets across the globe is three times higher than during the 2002-03 severe acute respiratory syndrome (SARS) epidemic and about 20 times higher than during the Ebola outbreak. The OECD estimated global growth in GDP at as little as 1.5 per cent for 2020, compared to 2.9 per cent in 2019, almost half of its previous estimate.
This is disrupting global production and supply chains without a date of resolution in sight. The World Trade Organization predicts that global trade growth would slow down to 4%, lower than the pre-pandemic trends.
Unsurprisingly, consumer confidence has also been hit. While confidence about an economy’s ability to recover differs greatly by geography, there is a definite shift to consumer spending on essentials and trading down to less expensive items, according to McKinsey.
Given these unprecedented circumstances, a lot of perceived business wisdom might have gone out of the window, and therefore it is crucial to leverage data to provide a reliable snapshot of the current situation that provides both grounded and immediate insight.
Here are few effective data analytics features that when properly implemented will provide valuable and decisive action points to strategize growth:
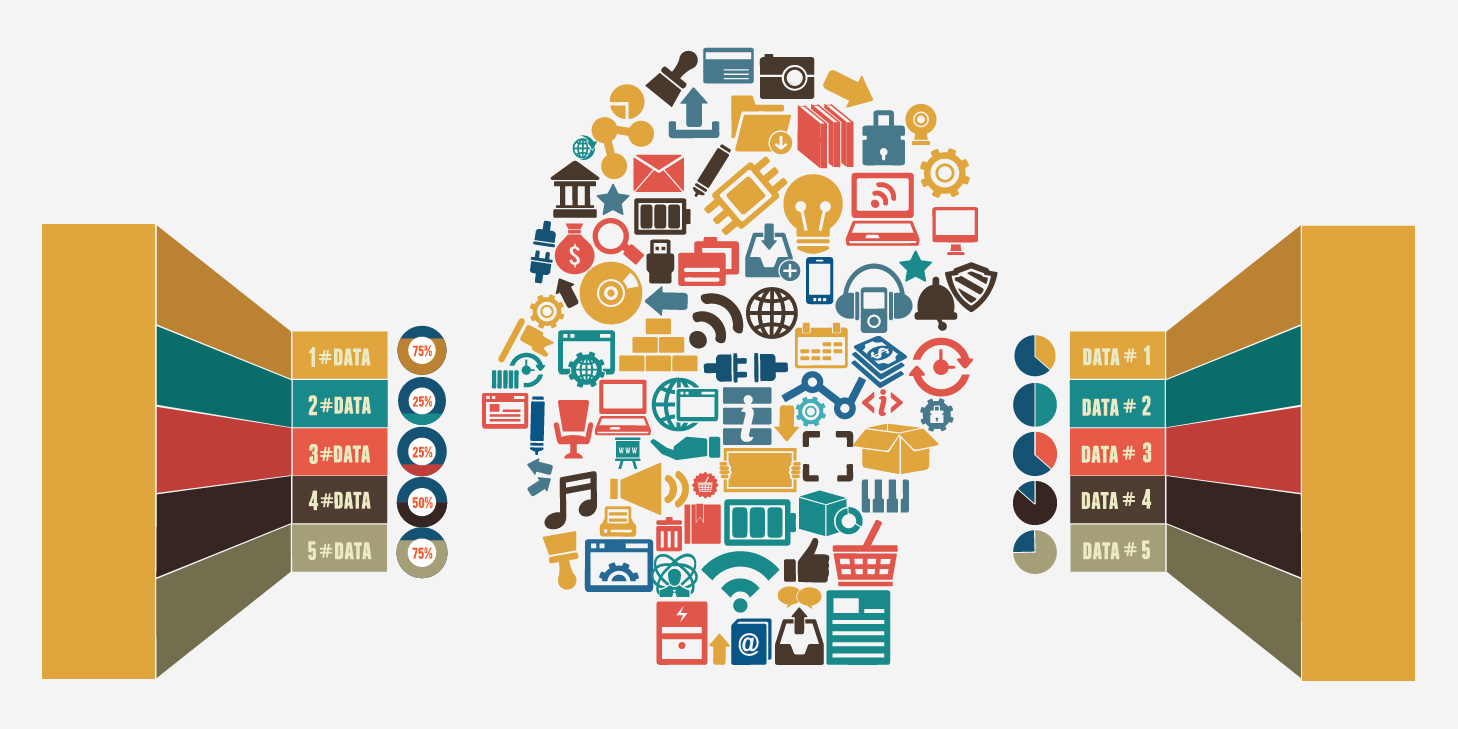
1. Product Recommendation Engines
According to a Mckinsey report, 35% of Amazon’s revenue is generated by its recommendation engine. These engines estimate what customer wants or needs and pair them with the right suggestions.
Product recommendation engines analyse customer behaviour and create personalized services for every single customer using their data. It simply predicts customer needs and provides appropriate recommendations.
Algorithms form the recommendation engine’s vital components. They scrutinize a large amount of customer data such as purchase history, items in cart, preferences and reviews and refines it into accurate recommendations. It provides the exact solution that any individual customer has been looking for.
The other key factor of recommendation engines is automation. The whole process from collecting data to analysing it and providing personalized recommendations to customers is automated. Companies save a huge amount of time, money and efforts by automating the entire process.
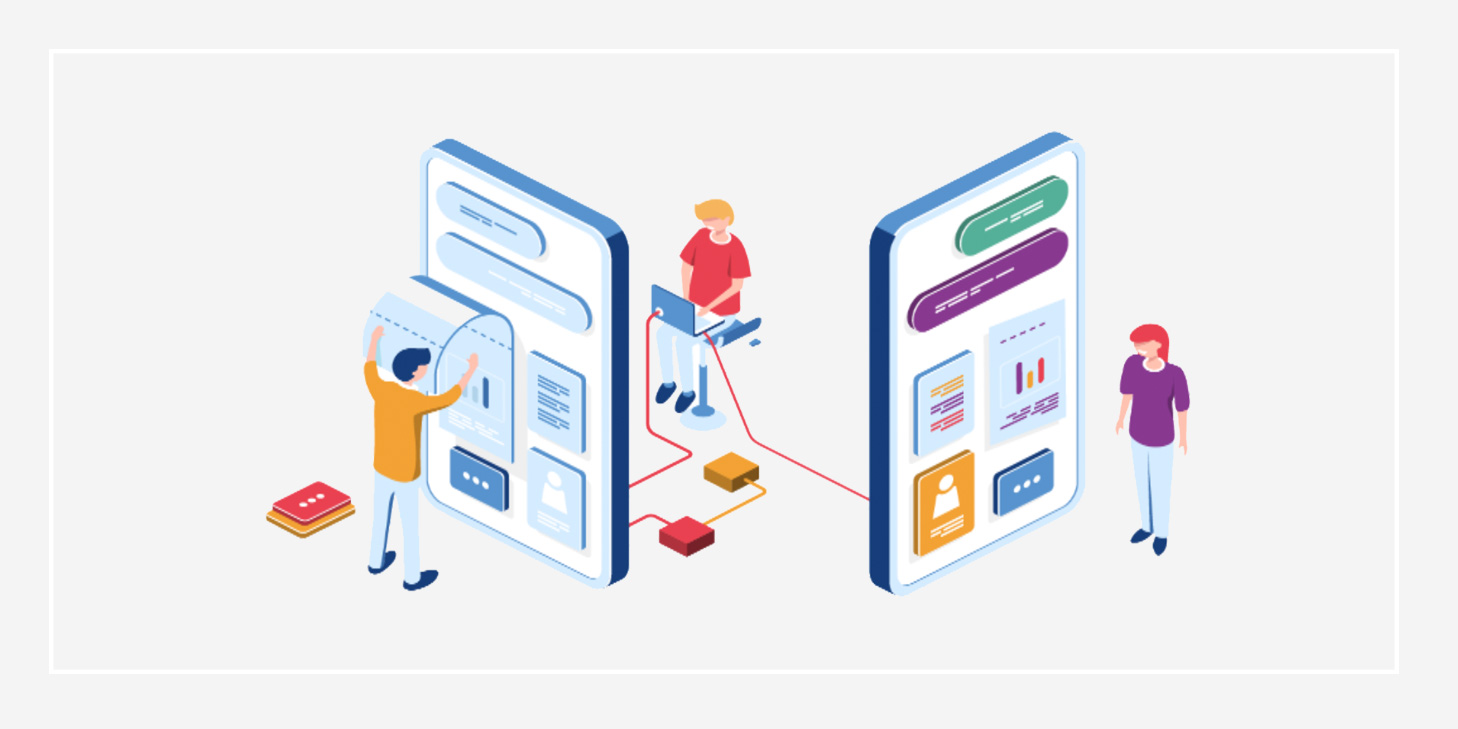
These engines use Machine Learning and Deep Learning algorithms to record their customers' consumption patterns and suggest them based on their behavioural analysis.
2. Market Basket Analysis
The second most celebrated feature is Market Basket Analysis. A key technique used by retailers to uncover hidden patterns and alliance between goods. Its purpose is to understand consumer behaviour by looking for associations between goods that are frequently bought together.
Market Basket Analysis uses Deep Learning algorithms, a subset of Machine Learning, and is modelled on the Association rule.
Association rule learning is an important concept of machine learning used by big retailers to check the dependency of one good on another for profitable measures.
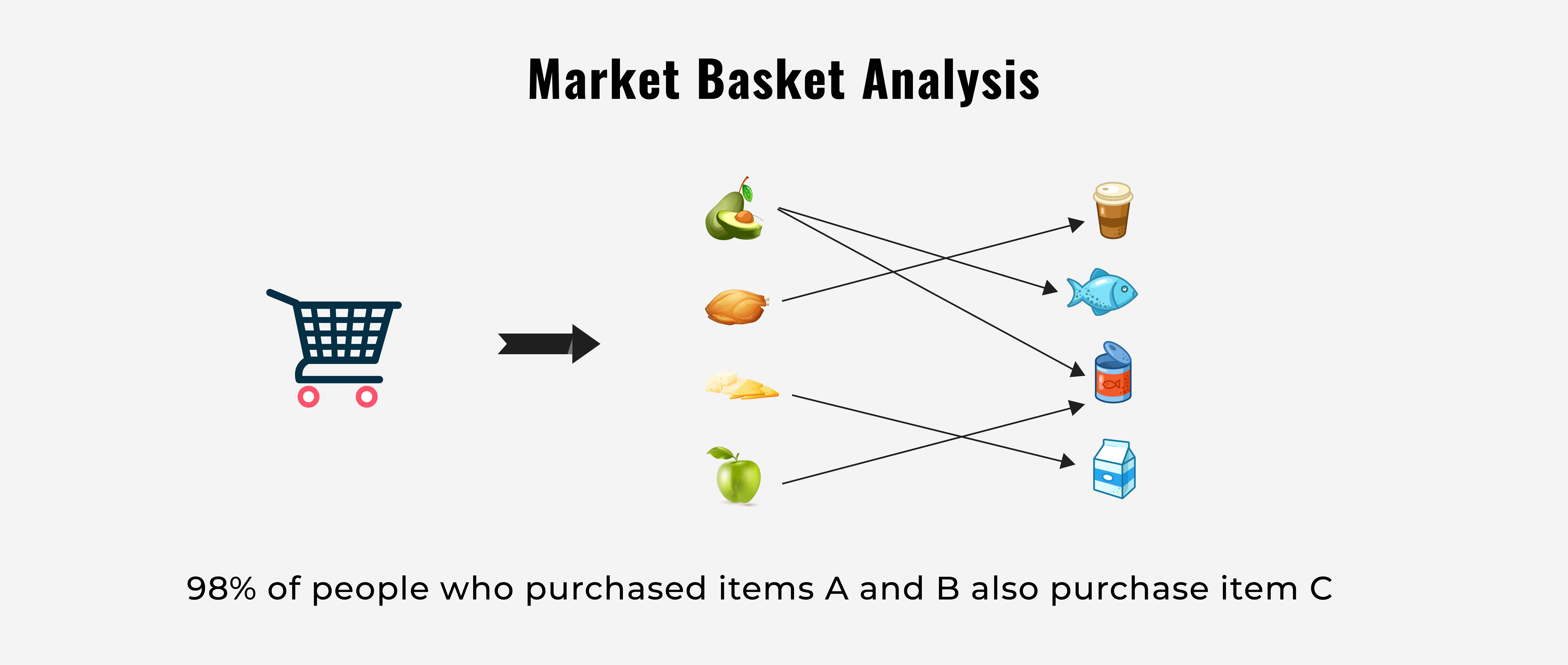
We can understand the implication of the association rule with an example:
Basket |
Product 1 |
Product 2 |
Product 3 |
1 |
Laptop |
Laptop Bag |
|
2 |
Laptop |
Laptop Bag |
Electric Kettle |
3 |
Laptop |
|
|
4 |
Electric Kettle |
Laptop |
Laptop Bag |
5 |
Laptop Bag |
Laptop |
|
6 |
Bottle |
|
|
7 |
Laptop Bag |
Laptop |
|
8 |
Laptop |
Bottle |
Laptop Bag |
The three reliability measures of association rule learning are: Support, Confidence and Lift.
a) Support
It indicates how often both the products are purchased together.
The number of transactions that include both the products ‘a’ and ‘b’ as a percentage of the total number of transactions.

The association rule Laptop => Laptop Bag has the support of 0.75 → Both products are bought together in 75% of the transactions.
b) Confidence
It indicates how often the association rule is true or how often both the products are bought together.
In mathematical term, the ratio of the number of transactions that include both the products ‘a’ and ‘b’ to the number of transactions that include the product ‘a’.

The association rule Laptop => Laptop Bag has the confidence of 0.86 → 86% of the times the customer buys a Laptop, Laptop Bag is bought as well.
c) Lift
It is the ratio of confidence to expected confidence.
Expected confidence is the probability that product ‘b’ is in the basket.
Purpose: The lift of a rule tells how strong the association within the rule is. In simpler words, how better a rule is at predicting the result than just assuming the result in the first place. Higher the lift values stronger the associations.
Lift = 1 → There is no relation between the purchase of the products.
Lift > 1 → The products are expected to be bought together.
Lift < 1 → The products are not expected to be bought together.
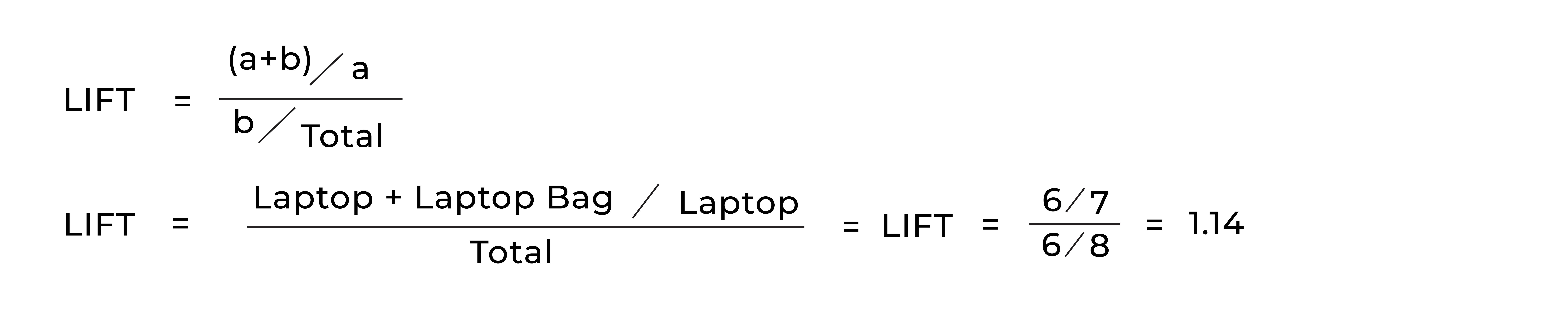
The association rule Laptop => Laptop Bag has a lift of 1.14 →
The probability of buying a laptop bag with the knowledge that a laptop is already present in the basket is much higher than the probability of buying a laptop bag without the knowledge about the presence of a laptop.
The presence of a laptop in the basket increases the probability of buying a laptop bag. Similarly, we can track transactions of different products, understand their relationships and use that insight to determine inventory, pricing and sales techniques.
3. Warranty Analytics
Warranty analytics provides exceptional insight into products in service and estimates ‘failure distribution’ based on data. It obtains a clearer perspective on the failure of products and customer satisfaction by analysing the information from products that fail.
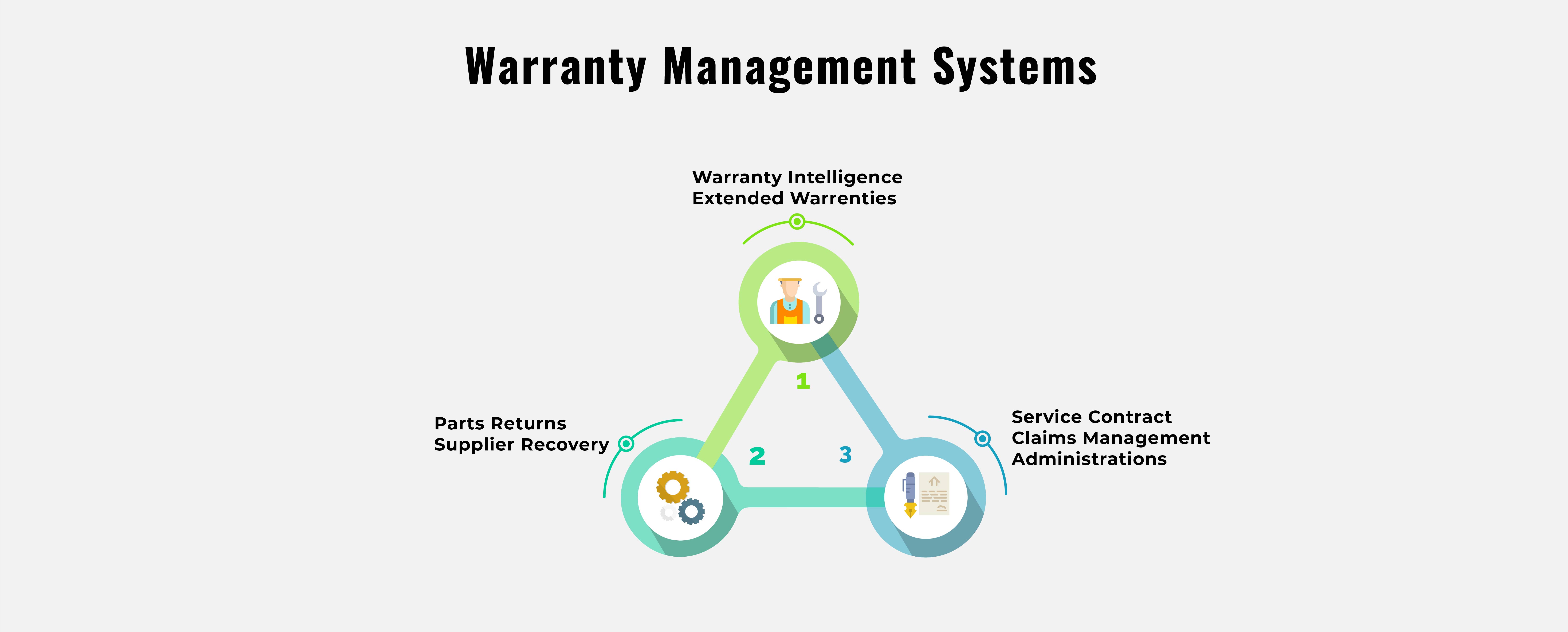
If a product is sent to a warranty repair in its fourth year of usage, it can be assumed that the product did not need any repair during the first three years.
A data visualization can be made using this information which shows failure rate over time for different systems and parts and how a product holds up over time. This analysed information helps retailers and manufactures to amend the warranty policies and improve customer satisfaction.
4. Price Optimization
Price optimization is a powerful feature that enables retailers to generate maximum profits by predicting the outcomes of various pricing strategies in an intensely competitive landscape. It integrates customer’s buying decision to meet retailers’ pricing needs. These engines are developed to predict changes in price by examining existing market data.
With varying demand at different points price optimization estimates how customers will respond if different prices are offered.
There are few concepts related to price optimization:
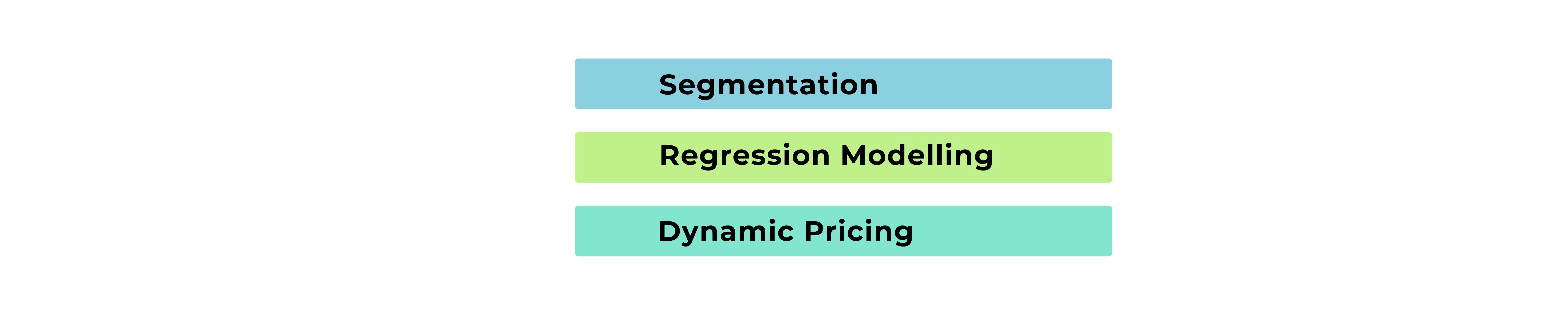
When it comes to price optimization, segmentation is one of the very powerful tools. Different people pay different prices for the same products even though the cost of offering is the same for each product.
After dividing the historical data based on some customer attributes other than prices into segments, data from each segment is fit to various price-response functions (Exponential, Weibull, Linear etc).
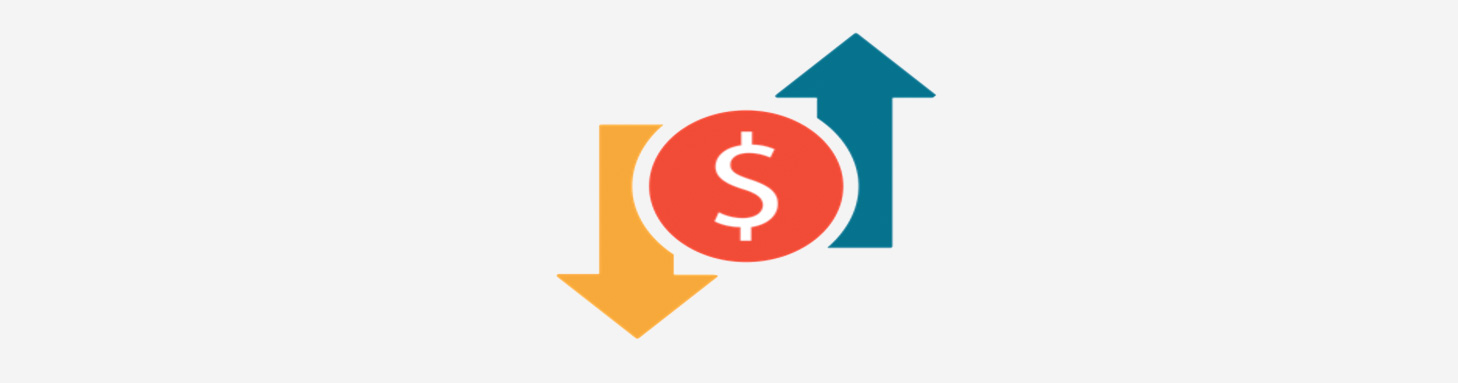
Dynamic Pricing enables businesses to set different prices for one product based on current market demands or how much a customer is willing to pay. For example, if you pay monthly for a subscription plan, say NETFLIX, your cost per month is often less if you pay annually for the same product. The results are then tested for the appropriate fit for each segment using Machine Learning algorithms.
5. Customer Sentiment Analysis
It is important to know how your customers feel about your products, services, or brands to improve customer experiences. This is where Customer Sentiment Analysis comes into the picture. Sentimental analysis gathers useful insights from textual contents from several social media platforms, blogs and comments.
Customer sentiment analysis is an automated process of discovering emotions through textual content, which helps understand customers' opinions.
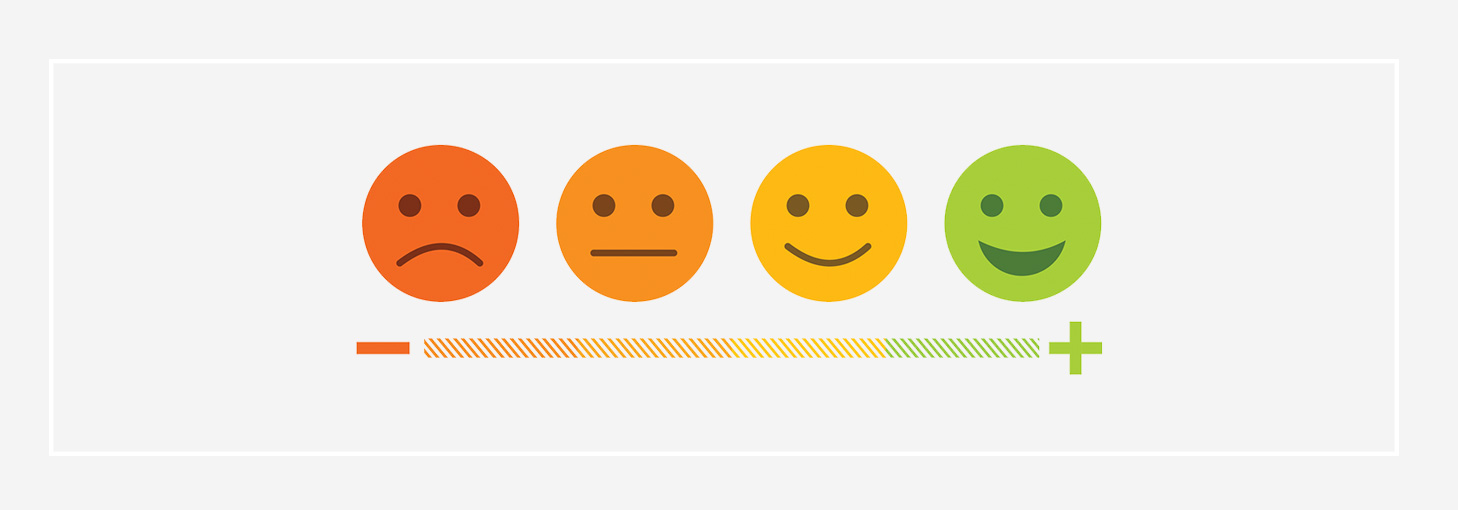
Sentiment Analysis uses Natural Language Processing (NLP), a key technique to study patterns. We can identify words and images bearing negative, positive inclination towards a brand and help companies boost their marketing strategy to meet consumer demands.
6. Demand Forecasting
Demand forecasting is the estimation of a probable future demand for a product or service. This technique helps to estimate demand for a product by analysing past demand and present market condition.
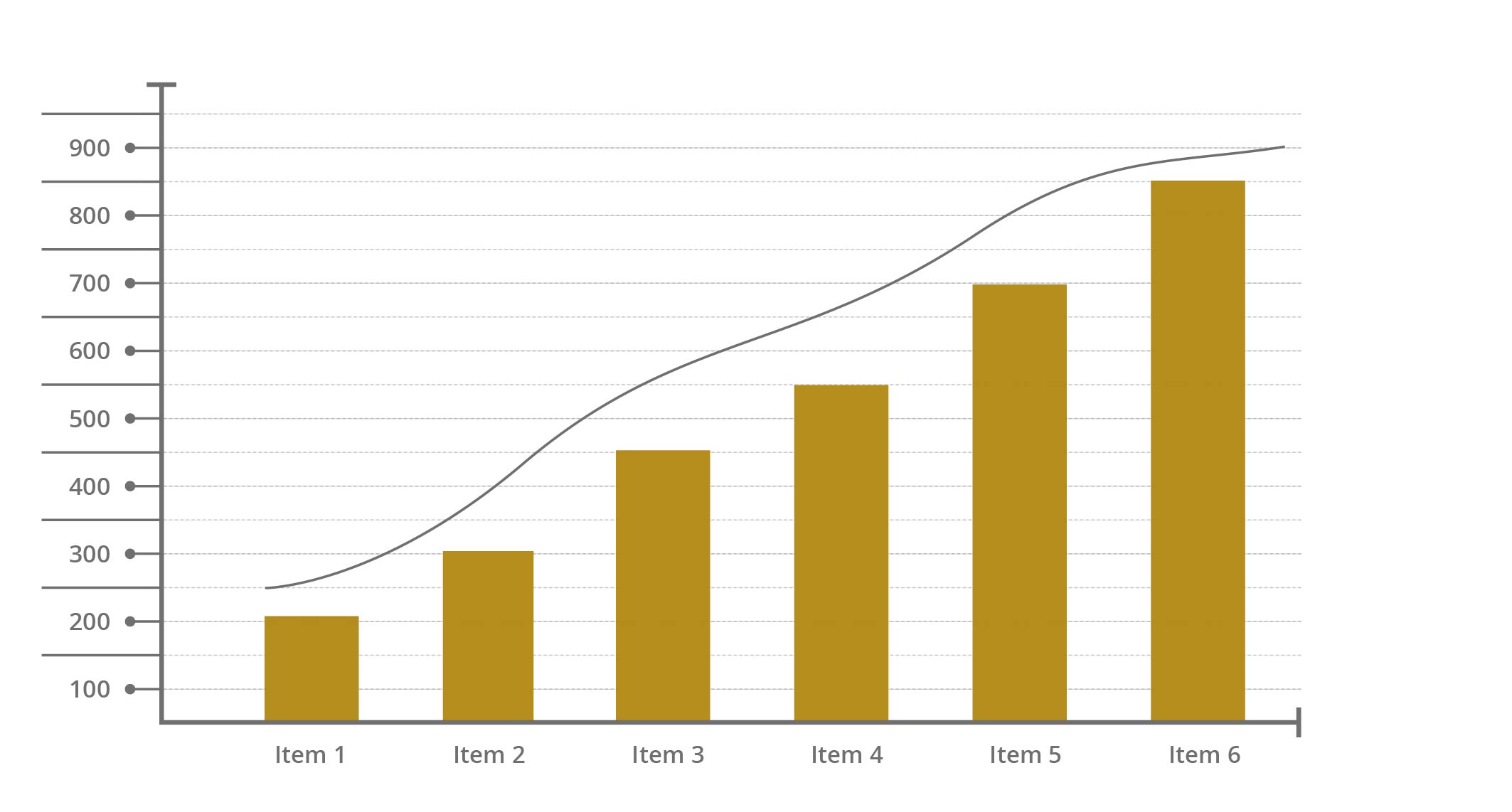
Several Machine Learning algorithms are available for demand forecasting. The choice of algorithm depends upon various factors: type, quality, and amount of data available. Even objective and period of forecasting, etc are determinant factors. Some commonly used approaches are:
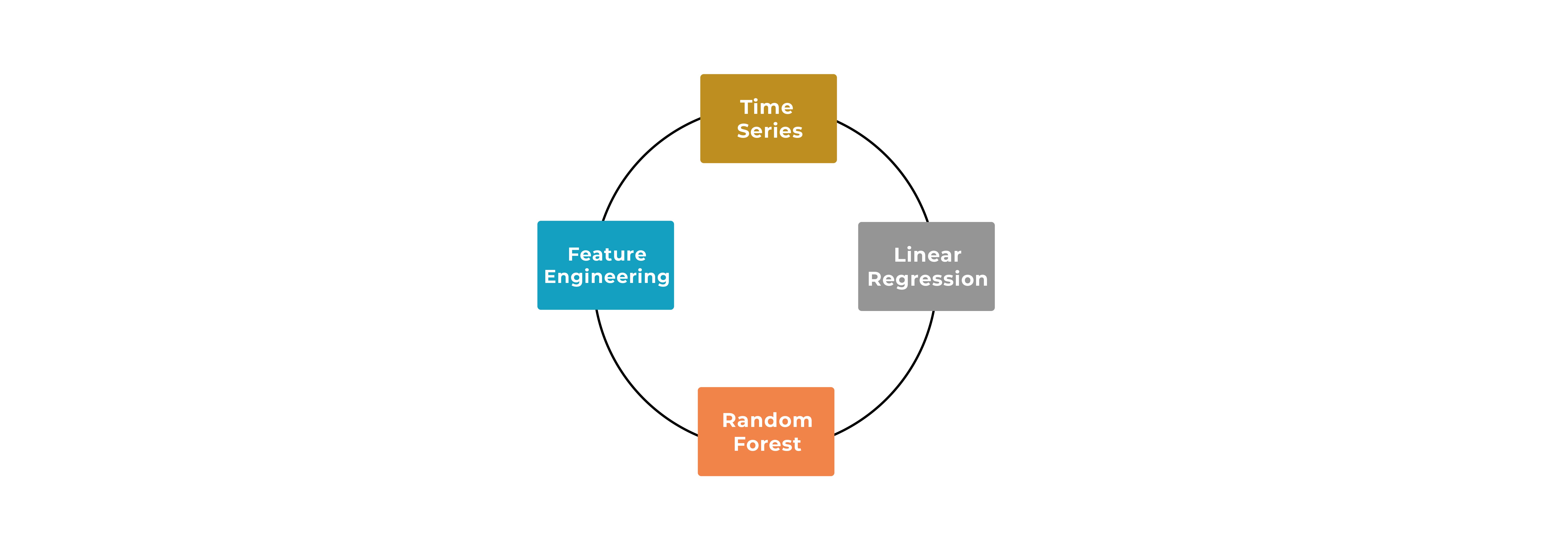
Demand Forecasting helps in: inventory management, marketing budget, advertisement investments and pricing plans. The beforehand knowledge of the demand for products and their fluctuations helps to plan better customer service.
These are some of the myriad applications of data analytics in the e-commerce retail industry and have prominently impacted the revenue streams and customer engagement for the e-commerce industry. A data-driven approach is a worthy investment. The business landscape is changing almost every day, but data can help you capture new customer trends much quicker than humans can. Businesses can leverage this knowledge to adapt to these new trends and optimize performance.
When dealing with uncertain situations, it is easy to be bleak. However, staying grounded with data will help you make rational business decisions during this most irrational of times.